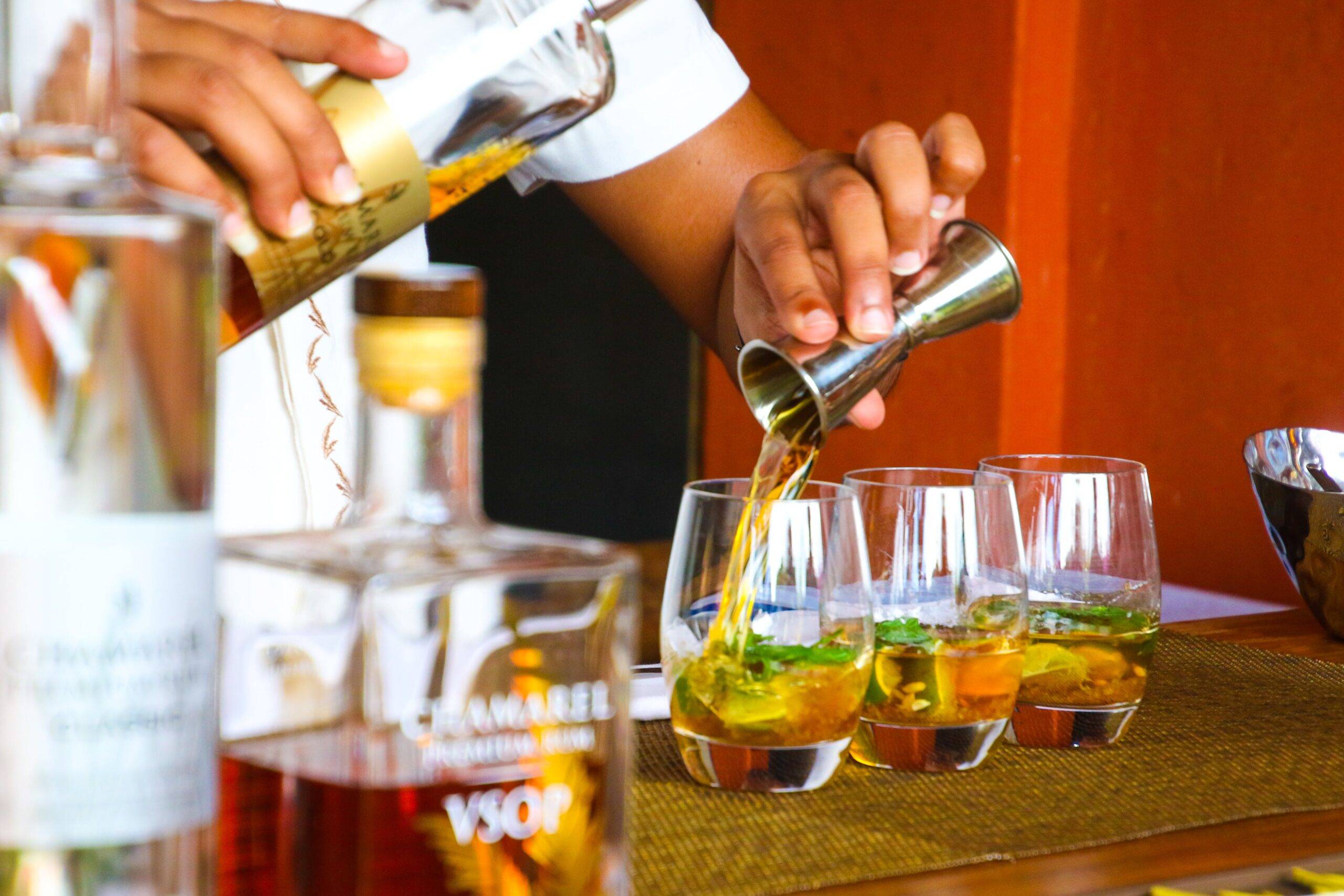
Image Source: Pexels
Are you a spirits enthusiast looking to explore the world of craft distilling? Look no further than the beautiful city of Canberra, where a vibrant distillery scene awaits you. Canberra is not only known for its political significance but is also home to a burgeoning craft spirits industry. With a wide array of distilleries offering unique and flavorful creations, Canberra has become a destination for spirit lovers seeking a taste of the local craft.
In this comprehensive guide, we will take you on a virtual tour of Canberra’s distilleries, providing you with all the information you need to plan your own Canberra distillery tour. Whether you’re a whiskey connoisseur, gin aficionado, or simply curious about the art of distilling, this guide will help you navigate the Canberra spirits landscape and discover the hidden gems that await.
Why Canberra for Distillery Tours?
When it comes to distillery tours, Canberra offers an experience like no other. The city’s distilleries combine traditional craftsmanship with innovative techniques to produce spirits that are both distinctive and exceptional in quality. Canberra’s cool climate and pristine natural surroundings provide the perfect conditions for growing the botanicals and grains used in crafting these fine spirits. Additionally, the city’s vibrant food and drink scene complements the distilleries, creating a rich tapestry of flavors and experiences for visitors.
The Canberra Distillery Scene: A Glimpse into Craft Spirits
Crafting Artisan Whiskies
The focus is on crafting artisan whiskies that embody the rich heritage and character of the Canberra region. Utilizing locally sourced grains and traditional distillation methods, some distilleries produce small-batch whiskies that are a testament to the region’s terroir. Take a guided tour of the distillery to witness the intricate process of mashing, fermentation, and distillation. Immerse yourself in the aromas of aging barrels and learn about the different types of casks used for maturation. After the tour, indulge in a tasting session where you can savor the complex flavors and nuances of their meticulously crafted whiskies.
Gin, the Spirit of Canberra
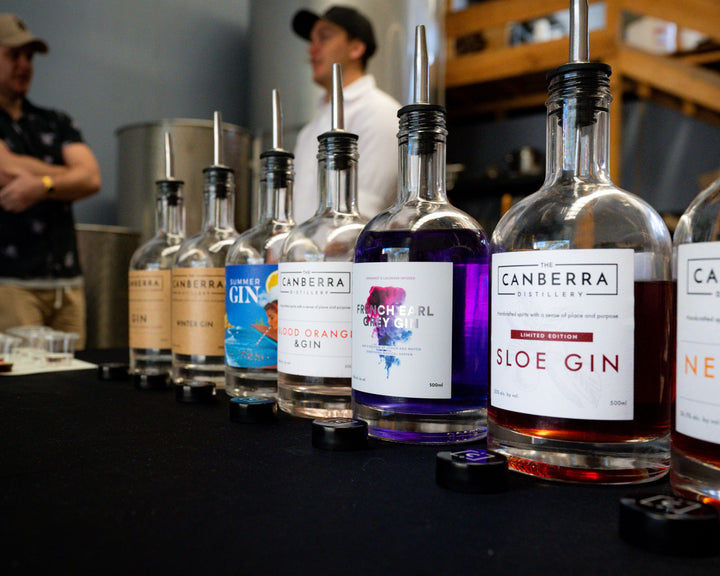
If gin is your spirit of choice, a local distillery is a must-visit on your Canberra distillery tour. This distillery specializes in handcrafted gins that capture the essence of the local botanicals. Take a stroll through their botanical garden and learn about the native plants and herbs used to infuse their gins with unique flavors. During the tour, you’ll have the opportunity to witness the distillation process and understand how each botanical contributes to the final product. Finish off your visit with a gin tasting, where you can explore the diverse range of flavors and aromas that make gins truly exceptional.
Vodka, Elegance in Every Sip
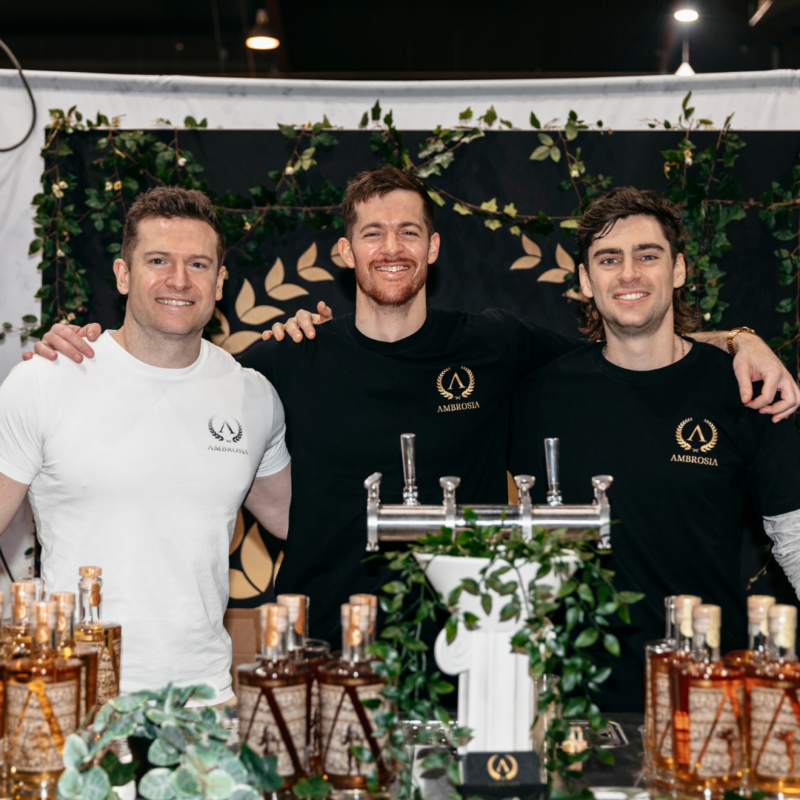
The focus is on crafting premium vodkas that are smooth, refined, and elegant. Using locally sourced grains and an attention to detail, many Canberra distilleries produce vodkas that are a testament to the art of distillation. During your visit, you’ll have the opportunity to witness the distillation process and learn about the importance of quality ingredients and precise techniques. The distillery tour culminates in a tasting session, where you can appreciate the purity and sophistication of their vodkas. From classic flavors to innovative infusions, the Canberra distillery offers a range of vodkas to suit every palate.
Planning Your Canberra Distillery Tour
Preparing for Your Tour
Before embarking on your Canberra distillery tour, it’s essential to plan ahead to make the most of your experience. Research the distilleries you wish to visit and check their opening hours and tour availability. Some distilleries may require advance booking, so be sure to secure your spot in advance. Additionally, consider arranging transportation to ensure a safe and enjoyable tour. Whether you choose to drive or opt for a guided tour, having a designated driver or transportation service is crucial, as alcohol tastings are an integral part of the distillery experience.
Distillery Tour Etiquette
When visiting distilleries, it’s important to respect their premises and follow any guidelines provided. Distilleries are working production facilities, and it’s essential to be mindful of safety regulations and any specific instructions given by the staff. Additionally, remember that distilleries are often small businesses, and purchasing their products is a great way to support their craft. Consider bringing home a bottle or two of your favorite spirits as a memento of your Canberra distillery tour.
Enhancing Your Experience: Wine and Brewery Tours
While exploring Canberra’s distilleries, why not expand your horizons and indulge in wine and brewery tours as well? Canberra is renowned for its exceptional cool-climate wines, and the region boasts numerous wineries offering tastings and cellar door experiences. Discover the diverse range of varietals, from crisp Rieslings to elegant Shiraz, and uncover the unique expression of the Canberra wine region. Additionally, Canberra’s flourishing craft beer scene is worth exploring, with local breweries showcasing their innovative and flavorful creations. Immerse yourself in the world of hops and malt, and discover the art of craft beer brewing.
The Art of Neat Pours vs Mixed Drinks
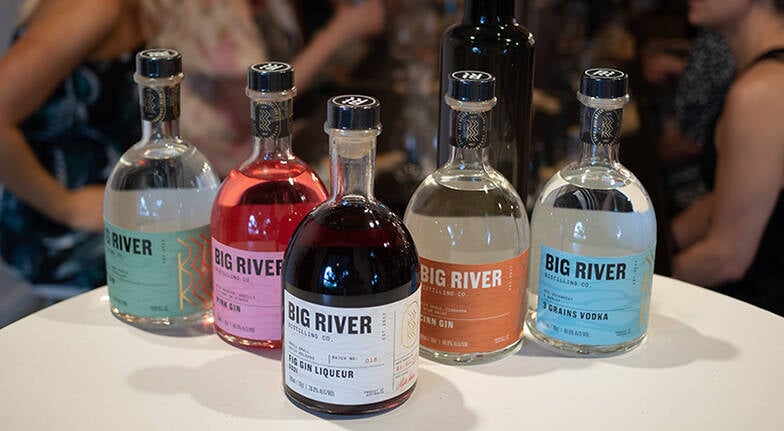
As you embark on your Canberra distillery tour, you may find yourself faced with the question of how to best enjoy the spirits you encounter. The debate between neat pours and mixed drinks is a subjective one, and personal preference plays a significant role in determining the best approach. Let’s delve into the nuances of each method to help you make an informed decision.
Neat Pours: Savoring the Essence
A neat pour refers to serving a spirit at room temperature, without any dilution or additional ingredients. This method allows you to fully appreciate the spirit’s flavors and aromas in their purest form. Neat pours are often preferred for spirits that are meant to be sipped slowly and savored, such as aged whiskies or premium vodkas. The absence of ice or mixers allows the spirit to shine, showcasing its complexity and depth. When enjoying a neat pour, take your time to explore the nuances of the spirit, allowing it to unfold on your palate and evoke a sensory journey.
Mixed Drinks: Unleashing Creativity
Mixed drinks offer an opportunity to explore the versatility of spirits and create unique flavor profiles. Cocktails allow for the fusion of different ingredients, such as fruits, bitters, and mixers, to complement and enhance the spirit’s base flavors. Canberra’s distilleries often provide cocktail recipes and recommendations, allowing you to experiment and showcase your mixology skills. Whether you prefer a classic cocktail or an innovative creation, mixed drinks offer a world of possibilities to tantalize your taste buds and unleash your creativity.
Finding Your Perfect Balance
The choice between neat pours and mixed drinks ultimately boils down to personal preference and the spirit at hand. Some spirits, such as aged whiskies, may be best enjoyed neat to fully appreciate their intricate flavors. Others, like gins or vodkas, lend themselves well to mixing, as their botanical or fruity notes can be beautifully enhanced by complementary ingredients. Feel free to experiment with both approaches during your Canberra distillery tour, allowing your taste buds to guide you towards your perfect balance of savoring neat pours and indulging in creative mixed drinks.
Embark on an Unforgettable Canberra Distillery Tour
As you embark on your Canberra distillery tour, prepare to be captivated by the artistry and craftsmanship behind each bottle of spirits. From the smooth elegance of vodkas to the complex depths of whiskies, Canberra’s distilleries offer a sensory experience like no other. Immerse yourself in the world of craft spirits, learn about the distillation process, and savor the flavors that embody the essence of Canberra.
Remember to plan ahead, respect the distillery premises, and consider exploring the region’s wine and brewery tours to enhance your Canberra experience. Whether you choose to enjoy neat pours or delve into the realm of mixed drinks, let your taste buds guide you on a journey of discovery through Canberra’s distillery scene. Cheers to unforgettable moments and the art of craft spirits!